What Animal Is Associated With Ebola Virus Outbreaks
Abstract
Ebola virus illness (EVD) is a contagious, severe and often lethal form of hemorrhagic fever in humans. The association of EVD outbreaks with forest clearance has been suggested previously but many aspects remained uncharacterized. We used remote sensing techniques to investigate the association between deforestation in time and space, with EVD outbreaks in Cardinal and West Africa. Favorability modeling, centered on 27 EVD outbreak sites and 280 comparable command sites, revealed that outbreaks located forth the limits of the rainforest biome were significantly associated with forest losses inside the previous 2 years. This association was strongest for closed forests (>83%), both intact and disturbed, of a range of tree heights (5–>nineteen chiliad). Our results propose that the increased probability of an EVD outbreak occurring in a site is linked to recent deforestation events, and that preventing the loss of forests could reduce the likelihood of hereafter outbreaks.
Introduction
Ebola virus illness (EVD) is a zoonosis that causes severe and often fatal haemorrhagic fever in humansone. EVD was first identified in Africa in 19762 and since then is estimated to take killed over thirteen,000 people3. Due to its associated high bloodshed and potential for contagion, EVD is viewed equally a global threat4. EVD is propagated past a group of filovirus species of the genus Ebolavirus (hereafter Ebola virus)1, but despite advances in understanding this zoonotic disease, the factors that trigger and maintain outbreaks remain elusive5. Such uncertainties impede the more accurate and effective prediction of outbreaks that would facilitate improved response or prevention6. Human activities may have promoted direct or indirect contact between humans and an creature reservoir of the virus7. Some suggest that the loss of forest tin can facilitate the spread of the disease to non-forest areas8,nine. The mechanism, although unknown, likely results from more frequent contact betwixt infected wild animals and humans. The latest outbreak in Guinea has been linked to contact with a bat colony, an issue that some have linked to forest loss10,11. However, the enabling part of forest loss in Ebola outbreaks seems difficult to reconcile with the upper Republic of guinea forests having been a dynamic mosaic of forest, savannah, and farmland for centuries, and that people in this region have been sympatric with bats, and other wood wildlife, throughout this history12. More generally, humansthirteen and swell apes14 have lived in close proximity to bats for millennia, thus information technology may be simplistic to claim that forest loss was sufficient to crusade the emergence of EVD and its repeated outbreaks.
Initial suggestions that deforestation increases zoonotic EVD outbreaks result from observations in vii West African EVD outbreak sites that revealed greater forest fragmentation in these locations than in their surroundingsfifteen. Quantitative analysis of the nexus between deforestation and the emergence of Ebola virus affliction has been recently undertaken past Rulli et al.16. Although this study showed that EVD outbreaks occurred mostly in hotspots of forest fragmentation, the spatio-temporal dynamics of this relationship was not considered. Here, using woods change remote sensing information17 and modeling nosotros investigate: (1) the spatio-temporal association between woods types and forest changes and the possibility of an outbreak, and (two) whether this association tin be extended to the whole distribution range of the Ebola virus in Westward and Central Africaeighteen.
In this study, our original hypothesis was that deforestation leads to increased contacts between humans and hosts or vulnerable mammals and thus leads to zoonotic outbreaks of EVD. The underlying assumption is that zoonotic manual would be more probable either because hunters would travel further (or therefore increase possible contacts) or considering in fragmented forests in that location tin can be an increased density of bats and other potential reservoirs of Ebola virus7,8,9. In order to test these relationships, nosotros investigated vegetation-cover changes within human populated areas where Ebola outbreaks have occurred and compared these to otherwise comparable localities where there have not been outbreaks. Out of the xl EVD outbreaks reported since 1976 we were able to focus on 27 sites where index cases (the first patient that indicates the existence of an EVD outbreak) were identified (Table S1) and for which big-scale deforestation data were available for the menses 2001–201417. We used spatial distribution modeling based on the Favorability Function19 to develop spatio-temporal distribution models using a gear up of predictor variables (Table S2) to discriminate between EVD outbreak locations and 280 control spatio-temporal locations (randomly selected sites containing human settlements but no recorded EVD outbreaks) (Figure S1). We assessed annual forest loss and fragmentation inside a xx-km radius buffer around each outbreak and non-outbreak site (See Methods); the buffer radius reflecting the distance hunters typically range from their villagestwenty,21 and thus defines the area where we assume zoonotic disease transmission could occur.
Results and Word
The spatio-temporal (STP) model detected a significant (χ2 = 55.286; p = ii.74 × 10−8) spatio-temporal trend in which 16 of the 17 EVD outbreaks during 2001–2005 were clustered around the Gabon-Republic of Congo edge, in the western Congo bowl. After 2006, viii of the nine outbreaks occurred in Uganda and eastern/southern Autonomous Democracy of Congo (DRC), and later 2013 in Due west Africa (Fig. 1A, Tables S3 and S4).
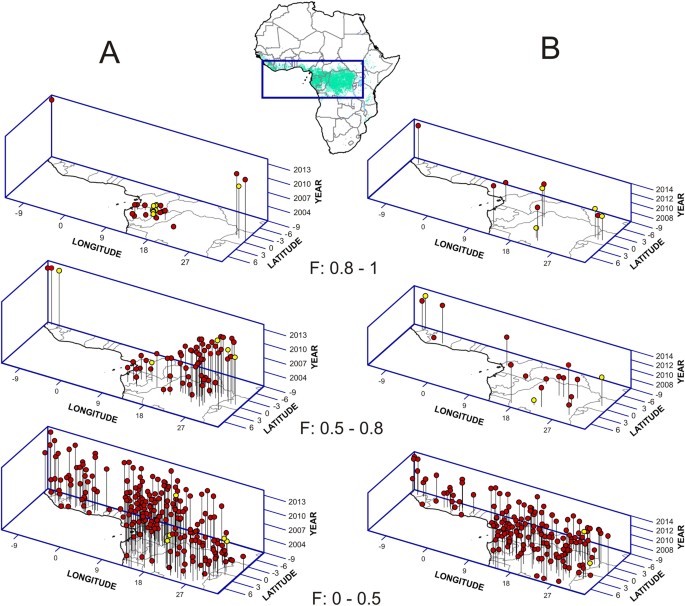
3D representations of two models of favorability (F) for the occurrence of EVD outbreaks. (A) Model describing the spatio-temporal pattern (STP) of outbreaks betwixt 2001 and 2014. (B) Model based on woods loss (FL) for the period 2006 and 2014. Yellowish points indicate outbreak locations; carmine points correspond random locations with no report of EVD outbreaks. Compared to random locations, favorability based on FL is significantly higher for seven of the ix outbreaks that occurred after 2005. The map of Africa shows the area represented by axes x and y; country borders are outlined in grey; the dark-green area represents rainforests (GlobCover version 2.ane database for 2005–2006, © ESA/ESA Globcover 2005 Project, led by MEDIAS-France/POSTEL).
Importantly, the 6 woods loss (FL) models only selected variables indicating forest changes less than 3 years prior to the outbreaks (Table S3). In all cases, college proportions of dumbo forest losses significantly favored subsequently EVD outbreaks. The most significant FL model (χ2 = 25.925; p = 0.000033), and with the highest capacity to discriminate between presences and absences of EVD outbreaks (AUC = 0.910), was that generated for the period 2006–2014 (Fig. 2, Tabular array S4). This model indicated favorable weather condition (F > 0.5) for seven of the ix EVD outbreaks in this menses —those in Republic of uganda, DRC and Republic of guinea, all close to the limits of the rainforest biome (Fig. 1B) — and low favorability (F < 0.5) for 89% of non-outbreak locations. The variable almost significantly associated with outbreaks, according to this model, was the proportion of 'not intact forest with dense comprehend (>83%) and alpine trees (>19 k)' lost in the buffer area the same yr as the outbreak occurred (Wald = 7.421; p = 0.006) (Figures S2 and S3, Tabular array S3). The densest awning cover (>83%) characterized iii out of the iv variables in the model. Even so, both intact and previously disturbed forests, and a broad range of tree heights (five–>19 m) were represented in the final model.
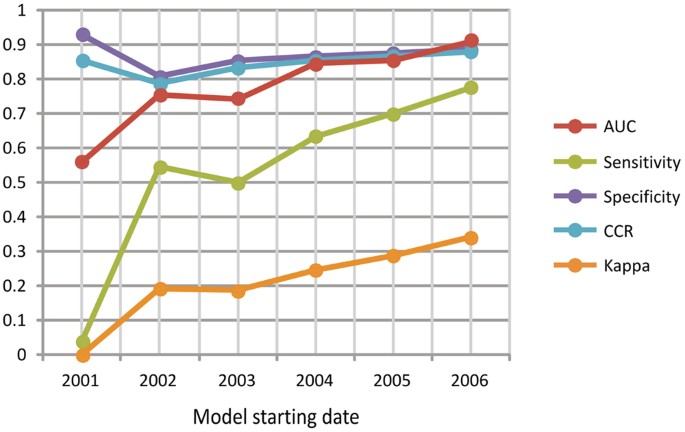
Capacity of discrimination (Expanse Under the Bend, AUC) and classification [sensitivity, specificity, Right Classification Rate (CCR) and Kappa] of the six models defining the favorability of the occurrence of EVD outbreaks due to woods loss (FL). The x-axis is the starting year of the fourth dimension period considered in the models.
The FL models that were run considering periods 2003–2014, 2004–2014 and 2005–2014 were sensitive to the 7 outbreaks explained by the 2006–2014 model, only they did not provide any evidence for a relation between forest loss and outbreaks prior to 2006. Only the FL model for 2002–2014 (χ2 = 20.vii; p = 0.000114) described favorable conditions for outbreaks that occurred before that year, although with less overall discrimination (AUC = 0.755) and nomenclature power than the 2006–2014 model (Fig. 2, Tabular array S4). Considering of this, both 2002–2014 and 2006–2014 time periods were further considered for variation partitioning analyses (See Supplementary Methods).
Significant basal spatial favorability (BSF) models were simply found for the periods 2001–2014, 2002–2014 and 2006–2014. In the ii erstwhile periods, at that place was a significant association (χ2 > five.000; p < 0.025) between favorability for the Ebola virus and outbreaks. This supports that differences in environmental potential for Ebola virus presence in the environment were related to the probability of occurrence of EVD outbreaks (Fig. 3, Table S3). All the same, this does not apply to the period 2006–2014, when outbreaks —which occurred forth the limits of the W and Primal African rainforest distribution range— were moderately associated to denser human populations (χtwo = ii.771; p = 0.096). Nevertheless, the bigotry and classification capacities of the BSF model between 2006 and 2014 were not as good (AUC = 0.812; sensitivity = 0.556; kappa = 0.175) as those based on wood loss (AUC = 0.910; sensitivity = 0.778; kappa = 0.341) (Tabular array S4).
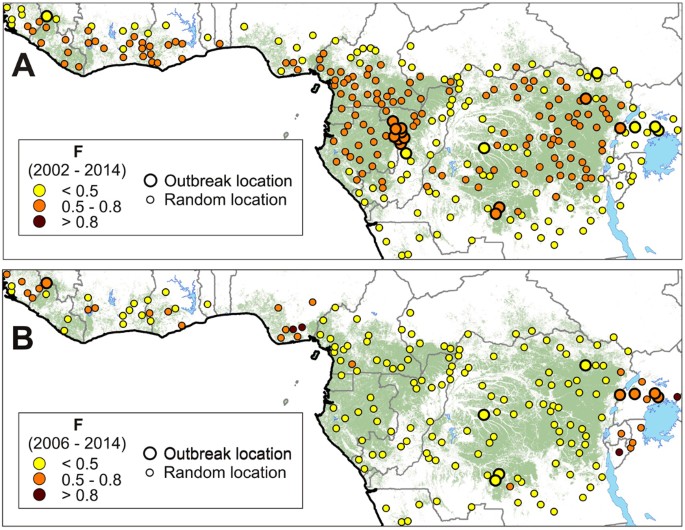
Models based on the basal spatial favorability (BSF) for EVD. Point colors indicate favorability (ranging 0–1). (A) The explanatory variable in the model for the menstruum 2002–2014 is the environmental/zoogeographical favorability for the occurrence of Ebola virus in the wild (12). (B) The explanatory variable in the model for the period 2006–2014 is the rural human population density. Maps were generated using ArcGIS 10.3 (http://desktop.arcgis.com/en/).
Variation sectionalization analyses regarding the complete Ebola virus surface areaeighteen (EVA) revealed that, in the period 2002–2014, the contribution made by woods loss (FL) alone to explain the variation in favorability for EVD outbreak was low (4.35%) compared with that made by the spatio-temporal pattern (STP) (82.44%) (Fig. 4). All the same, the importance of forest loss increased dramatically to 26.90% for the menses 2006–2014. In the surface area within the range of favorability values overlapping with EVD outbreaks (EVDA), forest loss lone accounted for half-dozen.88% of the variation (compared with 72.86% for the STP) in 2002–2014, but increased to 59.93% (vs. only 6.49% for the STP) in 2006–2014. From 2006 to 2014, the basal spatial favorability (BSF) explained a low proportion of the EVD outbreaks in EVA (four.74%), while gaining relevance in EVDA (12.95%), simply fifty-fifty then, forest loss alone was four.6 times more than relevant (59.93%) than the contribution of BSF alone.
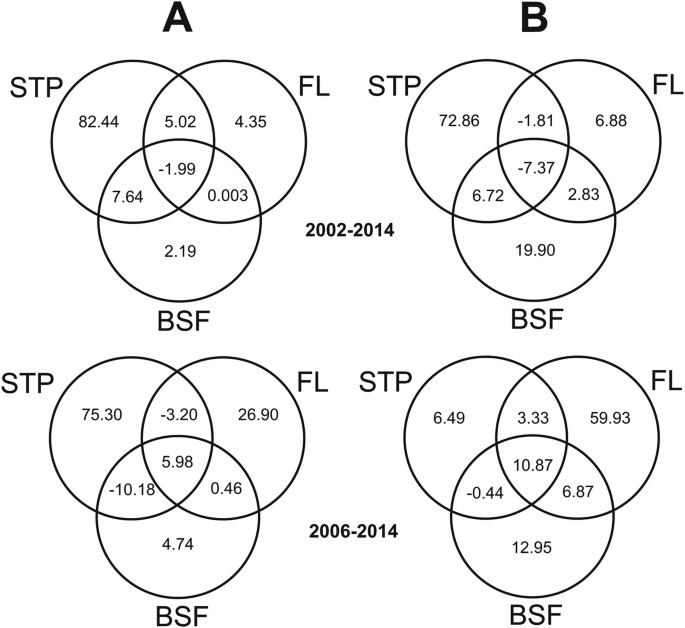
Venn diagrams displaying the results of variation partitioning analyses of a model combining spatio-temporal pattern (STP), wood loss (FL) and basal spatial favorability (BSF) for EVD outbreaks, for the periods 2002–2014 and 2006–2014. (A) Analysis focused on the complete Ebola-virus surface area (EVA)18. (B) Analysis focused on the range of favorability values overlapping with EVD outbreaks (EVDA).
Our study independently supports contempo findings of an association betwixt EVD outbreak locations and forest loss after 200416. In add-on, the spatio-temporal approach and the inclusion of all outbreaks after than 2000 revealed that: (i) the EVD outbreak-forest loss link is simply pregnant around the limits of the West and Fundamental African rainforest biome and excludes other EVD areas in the western Congo basin; (2) there is a time lag between forest loss and EVD outbreaks of two years; and (iii) the loss of dumbo forests, principally those with >83% canopy cover, is an important gene. We also bear witness that zoonotic EVD outbreaks appear in areas where human population density is high and where the virus has favorable conditions, just the relative importance of forest loss is partially (>60%) independent of these factors (Fig. 4).
The coupling between EVD outbreaks and forest loss in the margins of the rainforest biome within the previous 2 years, highlighted in our study, has profound implications. A plausible explanation is that contact between humans and infected wild animals increases dramatically subsequently the removal of wood. Such an outcome has been previously suggested22,23, and while our results strongly back up such an interpretation, they besides indicate that the changes are non sustained beyond two years. A multifariousness of ecological descriptors (e.g. species richness) are affected soon afterwards forest fragmentation24, and the factors promoting the emergence of the Ebola virus (host range, reservoir species, apportionment in nature) are all the same unknown5,21. Woods loss disrupts animal movements and local densities, and thus influences their interactions and the potential for a pathogen to be transmitted betwixt individuals and across species —though for Ebola such mechanisms remain theoretical. Regardless of whether or non fruit bats are important reservoirs of Ebola virus6, these animals are evidently involved in the virus' ecology25,26. Deforestation influences fruit bat motility and abundance23,27,28, and the composition, abundance and behaviors of the wider mammal fauna is influenced past timber cutting and disturbance29. Thus, forest loss and fragmentation could favor the combination of ecological events that are required for viral emergence. Interestingly, our results, which are not limited to tall intact one-time growth forests, highlight the clan between EVD outbreaks and shut-canopy forests.
EVD outbreaks may increase in the coming decades. Homo population growth, and greater penetration into Africa'southward remaining forests will be coupled with the proliferation of potential reservoir species as urbanization, agronomics and deforestation intensifyxxx,31,32. Greater population mobility via improved roads and air access increases the risks of an undetected EVD outbreak becoming a pandemic. A rapid response to whatsoever outbreak is fundamental to reducing contamination and reducing mortality, simply increased preparation and vigilance are needed to diminish the risk. The challenge, withal, is enormous. The vast and still relatively inaccessible areas involved, aslope the limited resources and manpower available, make it clear that priority setting will better guide monitoring and ensure preparedness. Wellness services in frontier areas require bolstering but the need for interdisciplinary approaches to improve our understanding of the ecology of the virus and its hosts, every bit nosotros suggest in our study, cannot exist neglected30. Our results provide indicators that could be useful for predicting where and when EVD outbreaks are more likely to occur. The data availability and image processing requirements of rapid predictions appear well inside electric current technical abilities, though the best way to update wood encompass classifications, or measures, requires further evaluation (to balance feasibility and utility). Such an approach would consider inhabited areas around the limits of the West and Fundamental African rainforest biome favorable for Ebola, and identify where dense encompass forests has been lost in the previous ii years. This predictive system would draw special attention to loftier-risk locations, which can exist updated and improved every bit data and concepts accelerate. For example, our analyses do not reveal why EVD outbreaks prior to 2005 occurred deep in the rainforest biome —within Commonwealth of Congo and Gabon— merely afterward shifted to more than transitional forests —in Uganda, DRC and Guinea. These regional shifts could result from inter-annual variation in rainfall across the continent33,34 and may reflect fruit availability and associated movements though more than data would be needed to clarify these putative relationships.
Prevention of EVD outbreaks is the ultimate goal. Accepting the inferred links to country cover equally causal implies that the chance of zoonotic EVD outbreaks tin be macerated by (1) reducing deforestation and (two) reducing human proximity and access to recently damaged forests (for two years). More generally, our results show that forest loss, similar EVD, should be seen as a major global wellness issue and should be managed and funded accordingly.
Methods
Vegetation Cover
We selected 27 locations where Ebola virus disease (EVD) outbreak "index cases" (i.e. likewise primary cases or patients zero, the initial identified patients in specific outbreaks assumed to upshot from zoonotic transmission) had occurred (Table S1), and 280 locations without outbreaks as control areas (run across criteria for buffer choice below in the section "Deforestation"). We evaluated vegetation-cover changes within a buffer area of radius twenty-km effectually each location selected. Based on past work in West and Central Africa we judged twenty km the distance that could typically be traveled by hunters20,21. Within these buffers we described vegetation cover changes as a set of dependent variables used in our models (Table S2). The use of non-overlapping buffers prevented the possible issues arising from spatial autocorrelation among the data.
Woods loss was estimated from data of vegetation cover changes contained in the University of Maryland's Global Wood Change (GFC) project17. The GFC dataset is a fourth dimension-series analysis of Landsat images characterizing forest extent and change at a 30-meter spatial resolution. This dataset reports annual forest extent, loss, and gain from the period 2001–2014. Because trees are simply defined equally vegetation taller than 5-m in height and with a awning cover of more than 25%, losses of natural forest or planted vegetation are non distinguishable using GFC data. Given this constraint, we applied the forest comprehend classification developed by Tyukavina et al.35, who stratified forests according to 7 distinct classes, based on canopy structure, as defined past percentage embrace, height, and intactness according to Potapov's et al.36 description of intact forest landscapes (IFL, divers as an unbroken surface area of natural ecosystems inside the zone of electric current wood extent, showing no signs of pregnant human activity, and large enough that all native biodiversity, including viable populations of wide-ranging species, could exist maintained)36:
- 1.
Forest with low cover (between 25–45% canopy cover).
- 2.
Forest with medium cover and short trees (45–83% canopy cover, five to 11-1000 superlative).
- 3.
Wood with medium cover and tall trees (between 45–83% canopy embrace, ≥11-chiliad acme).
- four.
Not IFL with dumbo embrace and short trees (>83% awning encompass, <19-thou height).
- five.
IFL with dense embrace and brusk copse (with no signs of human disturbance, >83% canopy encompass, <19-m acme).
- 6.
Not IFL with dumbo cover and tall trees (>83% canopy embrace, ≥19-chiliad height).
- 7.
IFL with dumbo embrace and alpine trees (pristine quondam-growth natural forests, >83% awning cover, ≥19-m height).
Wood expanse loss inside each 20-km radius buffer area was estimated annually for the period 2001–2014. This estimation was made in ha (i.e. accented forest loss, AFL), and too in pct respect to yr 2000 (i.eastward. relative forest loss, RFL). In social club to characterize the type of forest to which quantified tree-losses were related, we made all calculations with reference to these forest strata:
- 1.
Total woods (sum of strata 1 to 7).
- 2.
Dumbo forest (sum of strata 4 to vii).
- three.
IFL (sum of strata 5 and 7).
- iv.
Every forest stratum (1 to seven) separately.
Nosotros developed a second set of variables to describe patterns of woods fragmentation (Table S2), again employing information from the Global Forest Alter (GFC) project17:
- 1.
Mean distance to forest edge (MDFE)24: To quantify the class of forest fragmentation, we calculated the average distance between every forested pixel inside each xx-km radius buffer area and its nearest not-forest pixel. This variable was conceived as a spatial, not temporal feature of landscape, then the calculations were referred to a unmarried time period, for which we selected yr 2001 (i.e. the date of the first EVD outbreak considered).
- ii.
Increased edge (IE)24: This variable describes the change in relative length of the limit between forest and non-forest. We followed three steps: (ane) calculate the length of forest edge in 2000 (i.e. the year before the report period) and in 2014 (i.e. the end of the report period); (ii) calculate the increase in length between 2000 and 2014 (i.e. length in 2014 minus length in 2000); (three) calculate the proportion of increase with respect to the length in 2000.
MDFE and IE were calculated for total wood, for dumbo woods and for IFL.
Deforestation
Our dataset included the locations and dates of 28 EVD outbreak "index cases" since 2001 (Tabular array S1). Two outbreaks in Ekata (Gabonese republic) occurred in the same year and were merged for our assay; thus, operatively, the number of outbreak locations considered was 27.
Deforestation data were bachelor from 2001 to 2014. Thus, given that our working hypothesis was that EVD outbreaks might occur as a consequence of deforestation, only cases within this menstruation were considered.
In the search for ecologically meaningful relationships between deforestation and outbreaks, nosotros needed to analyze events inside both spatial and temporal contexts. We examined the geographic overlap of deforestation and EVD outbreaks and the time-calibration involved. Six time lags after deforestation events were examined: 0 to 5 years. As the outbreaks considered in the study included all alphabetize cases between 2001 and 2014, information technology was not possible to examine time lags higher than 0 in the outbreaks of 2001, time lags higher than ane in those of 2001 and 2002, and so on until 2006, for which fourth dimension lags from 0 to v years were available in the information gear up. Considering of this, the associations between outbreaks and forest loss were investigated past means of six temporally nested analyses, comprising from 9 to 14 years, all of them ending in 2014 (Table S5).
For our comparisons we selected 280 control locations where outbreaks have never occurred (Figure S1). All these locations were assigned random coordinates in three axes: breadth, longitude and time, considering the flow 2001 and 2014. The geographical context was W and Central Africa.
Locations where EVD outbreaks accept occurred, as well as the 280 randomly selected non-outbreak control locations were assessed for forest embrace alter. The random choice of not-outbreak locations was, however, subject to the following constraints:
- 1.
All such locations were favorable for Ebola virus in wild fauna18.
- 2.
A human population was present, according to at to the lowest degree 1 of the following sources:
-
Global Rural-Urban Mapping Project, Version one (GRUMPv1), Settlement Points (Palisades, NY: NASA Socioeconomic Information and Applications Centre SEDAC) http://dx.doi.org/x.7927/H4M906KR.
-
Interactive Wood Atlas of Cameroon, Car, Congo, DRC, Equatorial guinea and Gabon, http://world wide web.wri.org/our-work/project/congo-basin-forests.
-
Country, Cities and Places GIS Shapefile Map Layers, http://world wide web.mapcruzin.com/.
-
The Humanitarian Information Exchange (HDX) https://data.hdx.rwlabs.org/, NGIS Land Files http://geonames.nga.mil/gns/html/namefiles.html.
Because all our EVD index cases were in rural areas, we restricted our selection of control sites to such context. For this aim, we excluded human populations less than 20-km far from urban areas as the MODIS 500-yard Map of Global Urban Extent defines them.
- three.
These locations were more 40 km far from each other and from EVD outbreak locations, so that 20-km radius buffers did not overlap.
The terminal set of locations in the analysis consisted of 27 circles around EVD outbreak sites, and another 280 similar areas corresponding to non-outbreak sites.
Modelling
The Favorability Office (F, whose range is 0–one) tin be defined by both equations 1 and 2 19,37:
$$F=\frac{\frac{P}{(1-P)}}{\frac{{n}_{1}}{{n}_{0}}+\frac{P}{(ane-P)}}$$
(one)
where P is the probability of occurrence of an EVD outbreak co-ordinate to the set of predictor variables included in the model; n ane is the number of EVD outbreaks considered in the model; north 0 is the number of randomly selected non-outbreak control locations considered in the model;
$$F=\frac{{e}^{y}}{\frac{{n}_{1}}{{n}_{0}}+{e}^{y}}$$
(2)
where e is the base of operations of natural logarithms; y is a linear combination of predictor variables defining P. Both P and y were calculated with logistic regression, using IBM-SPSS Statistics 23. To avoid the inclusion of redundant variables in a model, and with some exception mentioned below, a forward stepwise procedure was performed according to the significance of Rao'southward38 score exam and the Akaike Information Criterion (AIC)39. This procedure identifies the most significant and informative elementary model (with only 1 predictor) and then nests it in increasingly more than complex models while guaranteeing that a variable was added only if it had an additional pregnant contribution to the previous data and that the new more than circuitous model was more efficient that the previous one. The parameters in the models were fitted by iteratively maximizing the log-likelihood of the model. Nosotros used χii tests to appraise the model's goodness of fit, and Wald tests to assess the contribution of every predictor variable in the model.
Past considering different sets of predictors (Table S2), nosotros modeled:
- 1)
The spatio-temporal pattern (STP) of EVD outbreak occurrences. EVD outbreaks could possess some geographical and temporal association (i.e. autocorrelation), depending on factors beyond the telescopic of this study (east.g. macroclimatic phenomena)33,40 which could drive to a clumped distribution41,42. We analyzed the STP in order to avoid errors in inference that can arise from this autocorrelation. We used the Favorability Role to build a STP model describing the distribution of EVD outbreaks co-ordinate to a set of variables resulting from combinations of breadth, longitude and the year of the outbreak (Table S2). Nosotros developed this method based on the trend surface approach41, but added time as a third dimension so that the model finally define a "trend book" describing the 3D-blueprint of EVD outbreak occurrences in Africa between 2001 and 2014. Variables in the STP model were recruited by backward stepwise selection; this enabled a ameliorate description of the spatio-temporal pattern by allowing model entry of a higher number of variables than with a forward stepwise approach.
- 2)
The spatio-temporal association between wood loss (FL) and EVD outbreaks. We run a set of FL models to define environmental favorability for the occurrence of EVD outbreaks in humans, according to changes happening in the forest immediately before the outbreak, and according to the fragmentation patterns observed. Nosotros applied the Favorability Function according to the set of forest-modify variables described above (see as well Table S2). In order to minimize Blazon-I errors arising from the large number of variables employed, we used Benjamini & Hochberg's43 Imitation Discovery Rate (FDR) procedure. Since six temporally nested FL models were produced, considering a unlike prepare of possible time lags between woods loss and EVD outbreaks (come across above), model comparisons were fabricated according to their bigotry (area under the ROC curve or AUC)44 and classification capacities (sensitivity, specificity, correct classification rate and Kappa)45; the model with the most successful assessment was selected.
- iii)
The clan of other ecology factors (i.e. "basal spatial favorability" or BSF) and EVD outbreaks. Even supposing that forest loss were involved in the occurrence of EVD outbreaks, index cases of EVD in humans could be bailiwick to two other basic requirements: (i) suitable environmental weather condition for the pathogen to proliferate, and (ii) a sufficient density of people to permit infection and contagion. Olivero et al.18 addressed the mapping of favorable areas for the Ebola virus in the wild as a function of climate (i.e. annual temperature range), terra-firme rain forest and some specific mammal types of distribution in Africa. Nosotros built a set up of BSF models by applying the Favorability Office to two predictor variables: (i) favorability for the occurrence of Ebola virus in the wild according to Olivero et al.xviii; and (ii) rural human population density as defined by a combination of the LandScan™ 2008 High Resolution Global Population Data Fix and the MODIS 500-m Map of Global Urban Extent. Six temporally nested BSF models were defined and compared with the aforementioned criteria every bit in the FL models (see higher up).
Finally, we estimated the relative contribution of each STP, FL and BSF in explaining where and when EVD outbreaks occur, by integrating their predictor variables into a single favorability model. We and then used variation partitioning analysis46, following the approach described by Muñoz et al.47 (see "Variation Sectionalization Analysis" in Supplementary Materials). Variation partitioning was applied twice separately: (1) considering the whole range of favorability values (0–1), representing the complete Ebola-virus surface area (EVA) as defined by Olivero et al.18; and (2) considering the area within the range of favorability values overlapping with EVD outbreaks (EVDA).
References
-
Kuhn, J. H. et al. Evaluation of perceived threat differences posed by filovirus variants. Biosecur. Bioterror. nine, 361–371 (2011).
-
Anonymous. Ebola haemorrhagic fever in Sudan, 1976. Balderdash. World Health Organ. 56, 247–270 (1978).
-
Centers for Disease Control and Prevention. Outbreaks Chronology: Ebola Virus Disease. Available at: https://www.cdc.gov/vhf/ebola/outbreaks/history/chronology.html (last accessed: August 2017) (2017).
-
Briand, S. et al. The international Ebola emergency. N. Engl. J. Med. 371, 1180–1183 (2014).
-
Olival, 1000. J. & Hayman, D. T. Filoviruses in bats: current noesis and future directions. Viruses 6, 1759–1788 (2014).
-
Wood, J. 50. et al. Ebola, bats and evidence-based policy: Informing Ebola Policy. EcoHealth 13, nine–11 (2015).
-
Bausch, D. G. & Schwarz, 50. Outbreak of Ebola Virus Illness in Republic of guinea: Where ecology meets economy. PLOS Negl. Trop. Dis. 8, e3056 (2014).
-
Wallace, R. M. et al. Did Ebola emerge in West Africa by a policy-driven phase modify in agroecology? Environ. Plann. A 46, 2533–2542 (2014).
-
Rogalski, M. A. et al. Human drivers of ecological and evolutionary dynamics in emerging and disappearing communicable diseases systems. Phil. Trans. R. Soc. B 372, https://doi.org/10.1098/rstb.2016.0043 (2017).
-
Sáez, A. et al. Investigating the zoonotic origin of the West African Ebola epidemic EOne thousandBOMol. Med. 7, 17–23 (2015).
-
Leendertz, S. A. J. et al. Assessing the evidence supporting fruit bats as the primary reservoirs for Ebola viruses. EcoHealth 13, xviii–25 (2016).
-
Fairhead, J. & Leach, Yard. Misreading the African Mural: Social club and Ecology in A Woods-Savanna Mosaic. (Cambridge Univer. Press 1996).
-
Leach, Thou. Ebola in Guinea –people, patterns, and puzzles. The Lancet Global Health Web log, http://globalhealth.thelancet.com/2014/04/03/ebola-republic of guinea-people-patterns-and-puzzles (2014).
-
Walsh, P. D. et al. Potential for Ebola transmission between gorilla and chimpanzee social groups. Am. Nat. 169, 684–689 (2007).
-
Environmental Resources Management (ERM). Ebola Virus Illness and Forest Fragmentation in Africa: A Written report past the ERM Foundation and the Environmental Foundation for Africa. The ERM Foundation, London (2015).
-
Rulli, One thousand. C. et al. The nexus betwixt forest fragmentation in Africa and Ebola virus disease outbreaks. Sci. Rep. 7, 41613 (2017).
-
Hansen, One thousand. C. et al. High-resolution global maps of 21st-century wood embrace change. Science 342, 850–853 (2013).
-
Olivero, J. et al. Mammalian biogeography and the Ebola virus inAfrica. Mamm. Rev. 47, 24–37 (2017).
-
Acevedo, P. & Real, R. Favourability: concept, distinctive characteristics and potential usefulness. Naturwissenschaften 99, 515–522 (2012).
-
Olivero, J. et al. Distribution and numbers of Pygmies in Central African forests. PloS ONE 11, e0144499 (2016).
-
Fa, J. E. et al. Correlates of bushmeat in markets and depletion of wild fauna. Conserv. Biol. 29, 805–815 (2015).
-
Wolfe, N. D. et al. Bushmeat hunting, deforestation, and prediction of zoonotic disease. Emerg. Infect. Dis. 11, 1822–1827 (2005).
-
Field, H. Eastward. Bats and emerging zoonoses: Henipaviruses and SARS. Zoonoses Public Health 56, 278–284 (2009).
-
Haddad, Northward. 1000. et al. Habitat fragmentation and its lasting impact on Earth'southward ecosystems. Sci. Adv. 1, e1500052 (2015).
-
Laupland, K. B. et al. Ebola: Lessons from the latest pandemic. Can. J. Infec. Dis. Med. Microbiol. 25, 128–129 (2014).
-
Leroy, E. M. et al. Human Ebola outbreak resulting from direct exposure to fruit bats in Luebo, Democratic Democracy of Congo, 2007. Vector Borne Zoonotic Dis. ix, 723–728 (2009).
-
Shafie, N. J. et al. Diversity design of bats at 2 contrasting habitat types along Kerian River, Perak, Malaysia. Trop. Life Sci. Res. 22, 13–22 (2011).
-
Castro, I. J. & Michalski, F. Effects of logging on bats in tropical forests. Nat. Conservação 12, 99–105 (2014).
-
Loveridge, R. et al. Motility behavior of native and invasive pocket-size mammals shows logging may facilitate invasion in a tropical rain forest. Biotropica 48, 373–380 (2016).
-
Allan, B. F. et al. Result of forest fragmentation on Lyme disease risk. Conserv. Biol. 17, 267–272 (2003).
-
Levi, T. et al. Does biodiversity protect humans confronting infectious affliction? Comment. Ecology 97, 536–546 (2016).
-
Morris, A. L. et al. Deforestation-driven food-spider web collapse linked to emerging tropical infectious illness, Mycobacterium ulcerans. Sci. Adv. two, e1600387 (2016).
-
Pinzon, J. Due east. Trigger events: enviroclimatic coupling of Ebola hemorrhagic fever outbreaks. Am. J. Trop. Med. Hyg. 71, 664–674 (2004).
-
Anyamba, A. et al. Climate-illness connections: Rift Valley Fever in Kenya. Cad. de Saúde Pública 17, 133–140 (2001).
-
Tyukavina, A. et al. Aboveground carbon loss in natural and managed tropical forests from 2000 to 2012. Environ. Res. Permit. 10, 074002 (2015).
-
Potapov, P. et al. Mapping the world's intact woods landscapes by remote sensing. Ecol. Soc. xiii, 51 (2008).
-
Real, R. et al. Obtaining environmental favourability functions from logistic regression. Environ. Ecol. Stat. xiii, 237–245 (2006).
-
Rao, C. R. Large sample tests of statistical hypotheses concerning several parameters with applications to problems of estimation. Math. Proc. Camb. Philos. Soc. 44, 50–57 (1948).
-
Akaike, H. A new wait at the statistical model identification. IEEE Trans. Autom. Control. nineteen, 716–723 (1974).
-
Schmidt, J. P. et al. Spatiotemporal fluctuations and triggers of Ebola virus spillover. Emerg. Infect. Dis. 23, 415–422 (2017).
-
Legendre, P. Spatial autocorrelation: Trouble or new image. Ecology 74, 1659–1673 (1993).
-
Southwood, T. R. E. & Henderson, P. A. Ecological Methods (Blackwell Scientific discipline Ltd, 2000).
-
Benjamini, Y. & Hochberg, Y. Decision-making the false discovery rate: a practical and powerful arroyo to multiple testing. J. R. Stat. Soc. Serial B 57, 289–300 (1995).
-
Lobo, J. M. et al. AUC: a misleading mensurate of the operation of predictive distribution models. Global Ecol. Biogeogr. 17, 145–151 (2008).
-
Fielding, A. H. & Bong, J. F. A review of methods for the assessment of prediction errors in conservation presence-absence models. Environ. Conserv. 24, 38–49 (1997).
-
Borcard et al. Partialling out the spatial component of ecological variation. Ecology 73, 1045–1055 (1992).
-
Muñoz, A. R. et al. Modelling the distribution of Bonelli's eagle in Spain: implications for conservation planning. Divers. Distrib. 11, 477–486 (2005).
Acknowledgements
This study was funded by UKAID and USAID. Jesús Olivero was partially supported by the project CGL2016-76747-R of the Spanish Ministerio de Economía, Industria y Competitividad and FEDER Funds. We are grateful various colleagues for continued discussions on the topic and in item Stephen M. Funk for his thorough comments on the manuscript.
Author data
Affiliations
Contributions
J.East.F., J.O., and R.Due north. planned the project. J.O., R.R., A.L.One thousand., Chiliad.A.F., M.A.S. and D.G. performed the analyses. J.E.F. and J.O. wrote the main manuscript text. D.P., J.South., S.K., S.A.L., J.Thou.V. and D.Southward. contributed to the grooming of the manuscript. All authors reviewed the manuscript.
Respective authors
Ethics declarations
Competing Interests
The authors declare that they have no competing interests.
Additional data
Publisher's note: Springer Nature remains neutral with regard to jurisdictional claims in published maps and institutional affiliations.
Electronic supplementary material
Rights and permissions
Open Access This article is licensed under a Creative Commons Attribution four.0 International License, which permits utilize, sharing, adaptation, distribution and reproduction in any medium or format, as long as you give appropriate credit to the original author(due south) and the source, provide a link to the Creative Commons license, and bespeak if changes were made. The images or other third party textile in this article are included in the article's Creative Commons license, unless indicated otherwise in a credit line to the material. If cloth is not included in the commodity'southward Creative Commons license and your intended employ is not permitted past statutory regulation or exceeds the permitted use, you volition need to obtain permission directly from the copyright holder. To view a copy of this license, visit http://creativecommons.org/licenses/by/iv.0/.
Reprints and Permissions
Most this article
Cite this article
Olivero, J., Fa, J.East., Existent, R. et al. Recent loss of airtight forests is associated with Ebola virus disease outbreaks. Sci Rep vii, 14291 (2017). https://doi.org/ten.1038/s41598-017-14727-nine
-
Received:
-
Accepted:
-
Published:
-
DOI : https://doi.org/10.1038/s41598-017-14727-9
Further reading
Comments
By submitting a annotate you agree to abide by our Terms and Community Guidelines. If you find something abusive or that does not comply with our terms or guidelines please flag it every bit inappropriate.
Source: https://www.nature.com/articles/s41598-017-14727-9
Posted by: stewartadvigul.blogspot.com
0 Response to "What Animal Is Associated With Ebola Virus Outbreaks"
Post a Comment